An analytics platform’s main objective is to assist business personnel in making data-driven choices. By converting company data into visuals and insights that function as a platform for the user to act, these platforms aid in the decision-making process.
What is data analytics?
There is no one definition of “data analytics,” but at its most basic, data analytics is the process of reviewing, cleansing, and transforming data to extract valuable insights and information. Data analytics can be used to support a wide range of business decision-making, from identifying new business opportunities to improving operational efficiency.
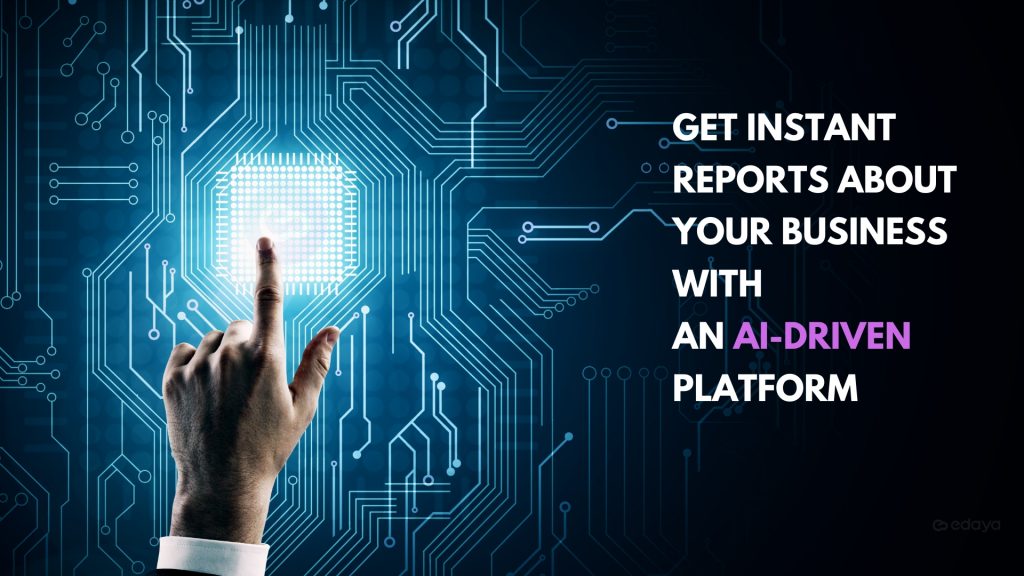
Evolution of analytics
1st stage of analytics: As obvious as it gets, this time period (which lasted till the early to middle of the millennium) exhibits the commencement, or rather the early years, of “Data Analytics.” In comparison to now, the 1950s were a time when there were clearly far less technical developments than there are now. That, however, did not prevent the businesses from trying to analyze data and subsequently derive usable information from it. The data was collected (offline) and manually analyzed by the firms. However, at this time, more effort was devoted to data collection and comparatively less to data analysis. As a result, a data analyst in this age was more of a technician, handling technology and gathering data. The task required a lot of physical labor, was laborious, and was supported by few data sources. The time it took to get the findings just made things more difficult.
2nd stage of analytics: an age that laid the path for improving the globe. This stage saw the phrase “big data” being established due to the vast amount of data present worldwide. This stage saw the expansion of analytics beyond huge corporations looking to maximize profits. Almost every company that dealt with digital infrastructure in some manner began implementing techniques for handling massive amounts of data and producing insightful conclusions. The drawbacks of the preceding period were improved upon during this one. Tools like OLAP, Data Mining, and other innovations that enhance technology were created in the early and middle 2000s. In light of this, “internet” skyrocketed in popularity and became the list of “Must haves,” not just for businesses but also for homes. The data analysts were in a much better position as a result of the development and maturation of technology as well as the availability of automated alternatives for handling data. Now, more than ever before, they could analyze data, patterns, etc. to draw conclusions and make suggestions. This time period gave us Google, PayPal, Amazon, and many more blessings. Additionally, the storage and processing of the growing amount of data presented challenges.
3rd stage of analytics: Smartphones, Facebook, Twitter, linked gadgets, etc. all become popular during this time period. In order to draw in customers, the businesses used stronger search engines as well as suggestions, recommendations, and analytics based on data. Here, we witnessed established market participants (firms) developing “Online Analytical Processing” systems. Companies became aware with a new category of databases called NoSQL after realizing they would also need to manage unstructured data. Machine learning models were employed for improved analytics, and new technologies were deployed for quicker processing. The era lasts until the late 2000s and the beginning of the 2010s. Data science has also shown to be a field with enormous potential. This opened the path for the need of data scientists.
4th stage of analytics: Despite the fact that this phase is still in its early stages, gathering data from several sources is not at all difficult. Organizations are ahead of the game when it comes to integrating cutting-edge automated decision-making systems that rely on cloud, big data, and predictive analytics. Massive streaming and intricate analytics are now available thanks to top cloud systems. It would not be unexpected to see what “data analytics” has in store for the future and how technologically advanced the world will become given how it has progressed over the years, from performing things manually to creating complex platforms and algorithms. preparing for bigger and more sophisticated technology to come!
Advanced analytics and future
Difference between analytics and advanced analytics:
While conventional analytics stick to basic calculations that only reveal what is occurring in the data without explaining why, advanced analytics uses techniques like machine learning and statistics to evaluate data.
Based on the user’s goal, advanced analytics chooses the best algorithm or model to utilize with the data. An advanced analytics platform, on the other hand, can employ forecasting methods to create forecasts for the future while also elucidating the underlying reasons of the original trend line. For instance, a standard analytics platform might provide straightforward trends based on historical data.
Therefore, by automating the creation of detailed and in-depth insights and suggestions, advanced analytics assists organization’s in making better decisions.
Techniques used in advanced analytics
Plenty of techniques used in advanced analytics here we discuss some of the techniques used in business intelligence to find better decisions.
Machine Learning
Machine learning is an AI method used in advanced analytics that makes use of algorithms to examine massive volumes of data. Machine learning may find similarities between many data sets to identify links that aren’t immediately obvious to the untrained eye.
To do this, the computer thoroughly and purposefully searches through the data, thus carrying out data analyst tasks far more quickly than a person could. As a result, the computer can examine an endless number of data combinations, making it typically more comprehensive than a person.
The machine can identify which information is most helpful for the user by experimenting with these data combinations. Machine learning specifically exhibits the quality of attention since it knows what is significant in the data and what the user should pay attention to.
By putting the power of machine learning in the hands of business users through advanced analytics, these users may quickly activate algorithms to resolve challenging business issues.
Natural Language Technology
The term “natural language technology” describes a computer’s capacity to comprehend and imitate human language.
Business intelligence is starting to use this capability more frequently, but advanced analytics shows complete semantic capabilities that are understood by business people.
Many consumers are already familiar with natural language processing (NLP), a type of semantic analysis, since they probably use Siri and other robotic assistants in daily life.
By speaking or typing a business objective or query into the platform, NLP in advanced analytics converts the user’s voice into a computer language, such as R or Python, to comprehend and respond to the inquiry.
On the other hand, NLG hasn’t yet established itself as a major force in the Business intelligence industry. NLG is a term for technology that generates output in human-intelligible language. NLG in advanced analytics takes the form of plain language insights that be written or spoken.
With the help of NLP and NLG, businesspeople may have a dialogue with their data in which they can ask a question and obtain an understandable response.
Data Visualization
Visualization is one of the most natural methods for people to comprehend their facts. Advanced analytics visualizations often refer to graphs and charts that reveal the story in the data.
The most pertinent information is directed toward the user through visuals when they are combined with natural language understanding.
Intelligent visualizations examine the response to a question to decide how to present the information most effectively. The computer may rationally decide, for instance, that metrics pertaining to particular states or areas should be shown on a map.
Furthermore, sophisticated analytics visualizations should be user-customizable so that they may be tailored to their specific requirements. When presented with similar questions, intelligent visualizations will remember these individual preferences, reducing the burden of repeated customization.
AI analytics, Faster Data Insights and Edaya
The use of AI in business analytics advances the automation of the data discovery process.
Business analysis may be more organized and outperformed by AI analytics, which also allows for more structured workforce allocations and fast data processing. in order for data analysts and business people to gain superior insights more quickly.
In the process of analyzing data, AI analytics displays complex human-like traits like learning and reasoning.
More about AI in hospitality AI and hospitality industry
A new generation of hospitality software platform called Edaya uses AI and its methods to enhance its features. And both hotel owners and visitors can use those extensively.
AI has a significant impact on Edaya, as shown by its own sentiment analysis. It is a unique review analysis approach to draw particular conclusions from each review by extracting the emotion, tone, intent from user reviews. It helps hoteliers to learn more about their customer perspective and make decisions based on qualitative feedback.
A sentiment analysis tool can help your team compile and report user comments more efficiently.
A sentiment analysis tool examines your input and automatically determines whether it is good, negative, or neutral instead to looking through each message and remark one at a time. The data is then combined and presented in charts or graphs that make evident the trends in your consumer feedback. This not only provides your team with precise information to work with, but it also offers your staff more time to complete additional activities as part of their regular workflow.
A key AI-enabled component of Edaya is its customized recommendation system, which directs visitors to the hotel’s numerous amenities including the spa and cuisine options. Also, it’s a kind of semantic similarity evaluation. Extraction of user preferences from text comments is followed by suggestions to restaurants, customization in accordance with user preferences, and recommendations to clients for meals.
The analyzed data is represented through data visualization methods.
For more or book a demo with Edaya, visit: edaya